Generative AI | Artificial Intelligence | Business | Conversational AI | Intelligent Automation | AWS | Oracle | DevOps | Innovation | Cloud Engineering | Data Engineering | Managed Services | Solution Architecture | Supply Chain | VAPT | Implementation | Jobs | Resume | CV | Scrum | Cloud Consulting | AI | Machine Learning | Cloud Computing | Azure | Technology | Business Intelligence | FMCG | Data Analytics | Configuration | Staffing | Recruitment | Agile | Operations | Business Consulting
Enhancing Logistics Efficiency with AI in Route Optimization
Read Time 7 mins | Written by: Praveen Gundala
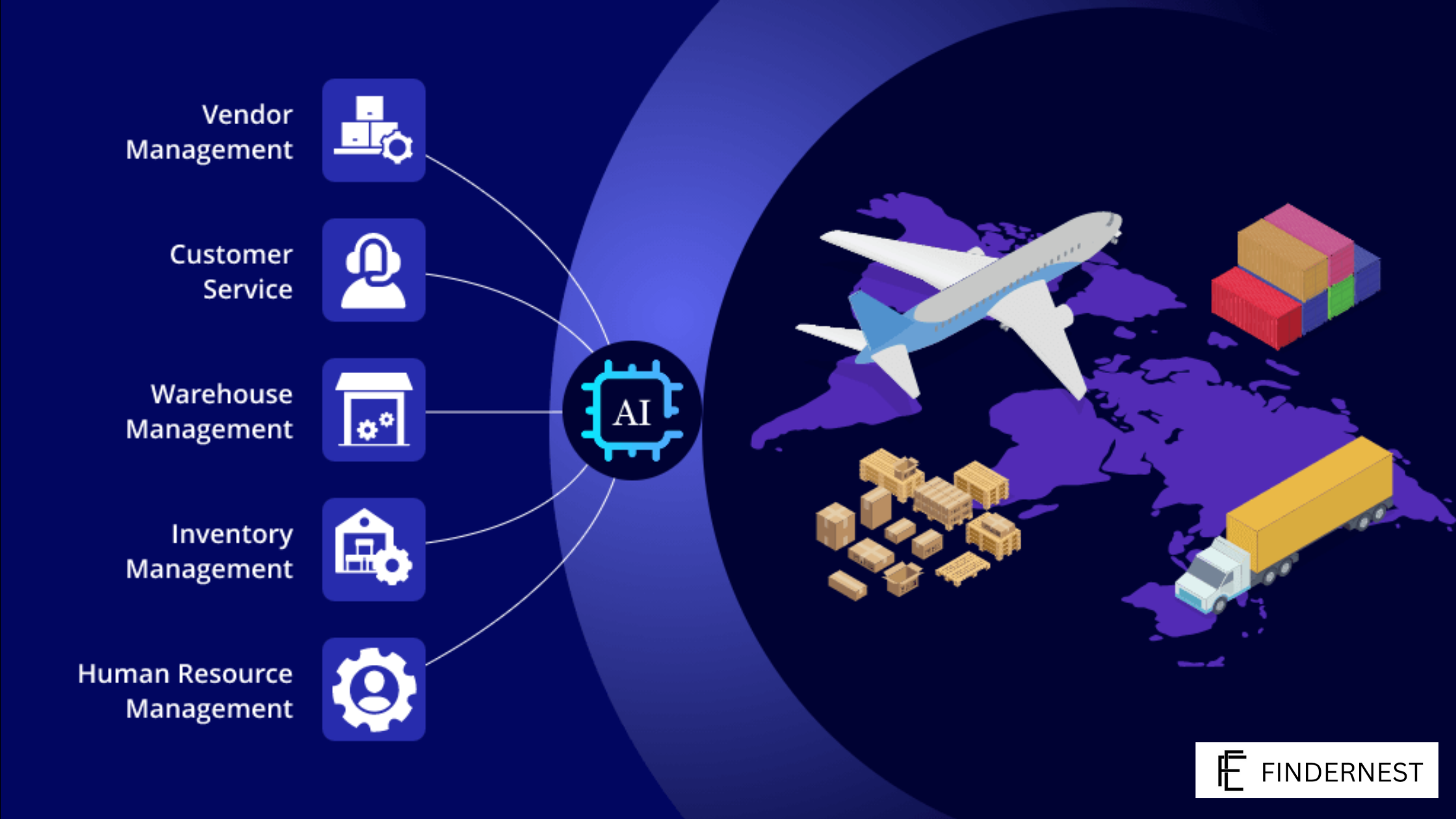
Discover how artificial intelligence is revolutionizing route optimization in the logistics industry, leading to increased efficiency and cost savings.
The Role of AI in Logistics
Artificial intelligence (AI) plays a crucial role in revolutionizing the logistics industry, particularly in the area of route optimization. By leveraging advanced algorithms and machine learning capabilities, AI enables logistics companies to optimize their supply chain operations, streamline delivery routes, and improve overall efficiency.
With AI, logistics companies can analyze vast amounts of data, including real-time traffic information, weather conditions, and customer demands, to make informed decisions about route planning. By considering various factors such as distance, time, fuel consumption, and vehicle capacity, AI algorithms can generate optimal routes that minimize costs and maximize efficiency.
Furthermore, AI-powered logistics systems can continuously learn and adapt based on historical data and real-time feedback. This allows companies to continually improve their route optimization strategies and respond effectively to changing market conditions and customer demands.
Overall, AI plays a transformative role in logistics by enabling companies to optimize their supply chain operations, reduce costs, and provide faster and more efficient delivery services.
Challenges in Traditional Route Optimization Methods
Traditional route optimization methods in the logistics industry often face several challenges that can hinder efficiency and cost savings. These challenges include:
1. Complexity: Traditional methods rely on manual calculations and heuristics, which can be time-consuming and prone to human errors. The complexity of logistics networks with multiple nodes, delivery points, and constraints makes it difficult to find optimal routes.
2. Lack of Real-Time Data: Traditional methods often lack access to real-time data such as traffic conditions, weather updates, and customer preferences. Without this information, it is challenging to make accurate route planning decisions.
3. Inability to Adapt: Traditional methods typically follow fixed, pre-determined routes that do not adapt well to dynamic changes in the logistics environment. They are unable to consider real-time events such as traffic congestions or road closures, resulting in inefficient routes and delays.
4. Limited Optimization Capability: Traditional methods often focus on optimizing individual routes rather than considering the entire supply chain network. This approach may lead to suboptimal overall performance and missed opportunities for cost savings.
By addressing these challenges, AI-powered route optimization systems offer significant improvements over traditional methods, enabling logistics companies to overcome limitations and achieve enhanced efficiency.
How do we implement Gen AI for logistics and route optimization?
Let's explore the application of generative AI in supply chain management to optimize routes and logistics.
By collecting data
The first step involves collecting relevant data, which can include past records connected to transportation, traffic patterns, climate, delivery schedules, and vehicle specifications. This collected data will serve as the training dataset for the generative AI model.
Training the Gen AI model
The next step involves training the generative AI model using the collected data to understand the various connections and variables that impact route optimization. This training process is crucial as it allows the model to delve deep into the intricacies of transportation efficiency. By employing advanced deep learning methods, such as neural networks, the AI model can not only detect patterns but also uncover hidden insights that traditional methods may overlook. Through this process, the model gains the ability to analyze historical trends, identify key factors influencing route optimization, and adapt to dynamic changes in the logistics environment. This deep learning approach enables the AI system to make informed decisions and generate optimized routes that are tailored to specific needs and constraints, ultimately leading to enhanced efficiency and cost savings for logistics companies.
Integrating real-time data
Once the model is trained, it is crucial to seamlessly integrate the real-time data feeds from the logistics company's system into the generative AI model. This integration not only includes up-to-the-minute traffic information and weather forecasts but also factors in any unforeseen events that could potentially impact travel routes and schedules. By incorporating this real-time data, the generative AI model is empowered to make dynamic and informed decisions, ensuring that routes are optimized in response to changing conditions instantaneously. This real-time data integration acts as a catalyst, propelling the AI system into action and enabling it to continuously adapt and refine its route optimization strategies for maximum efficiency and effectiveness in logistics operations.
Dynamic route optimization
The generative AI model meticulously analyzes the routes for each truck in the fleet, taking into consideration a plethora of factors such as real-time traffic congestion, delivery schedules, vehicle capacities, fluctuating fuel prices, and unexpected road closures. By dynamically adjusting the routes based on these critical variables, the AI system ensures that deliveries are not only made on time but also in the most cost-effective manner possible. This level of precision and adaptability guarantees that logistics companies can meet their delivery commitments efficiently and effectively, ultimately enhancing customer satisfaction and optimizing operational performance.
Multi-objective optimization
Leveraging a multi-objective optimization approach, the Gen AI model adeptly aligns conflicting objectives within the logistics landscape. Whether it's the challenge of reducing transportation costs, ramping up delivery speed, or meeting strict customer timeframes, the Gen AI model skillfully crafts a collection of Pareto-optimal solutions, that strike the perfect balance between these diverse goals.
Scalability and flexibility
As the logistics operation expands, the generative AI model is designed to seamlessly scale up. With its inherent scalability and flexibility, the model can effortlessly adjust to accommodate varying needs. It adeptly manages multiple trucks, shipments, and route optimizations while remaining responsive to supply chain dynamics like new delivery locations, shifts in demand patterns, and other evolving factors.
Continuously learns and improves itself
The AI model is designed to constantly evolve and improve its capabilities by assimilating new insights and information gleaned from user feedback and real-time data updates. Through this iterative process, the model refines its route planning prowess, incorporating fresh knowledge and adjusting to changing circumstances and requirements. This ongoing cycle of learning allows the model to continually enhance its knowledge base and refine its optimization strategies, ensuring optimal performance and adaptability in logistics operations.
Benefits of AI in Route Optimization
The integration of AI in route optimization brings several benefits to the logistics industry. These benefits include:
1. Cost Savings: By optimizing routes, AI-powered systems can minimize fuel consumption, reduce vehicle wear and tear, and optimize resource allocation. This leads to significant cost savings for logistics companies.
2. Increased Efficiency: AI algorithms can generate optimized routes that consider various factors such as distance, time, traffic conditions, and delivery constraints. This leads to improved efficiency in terms of faster delivery times, reduced idle time, and better resource utilization.
3. Enhanced Customer Satisfaction: AI-powered route optimization enables logistics companies to provide accurate delivery time estimates, optimize delivery schedules, and improve overall service quality. This results in higher customer satisfaction and loyalty.
4. Flexibility and Adaptability: AI systems can adapt to real-time events and dynamically adjust routes based on changing conditions. This ensures that logistics operations remain agile and responsive, even in the face of unexpected disruptions or delays.
Overall, the benefits of AI in route optimization contribute to the overall competitiveness and success of logistics companies by improving operational efficiency and customer satisfaction.
Case Studies: Successful Implementation of AI in Logistics
Several real-world case studies demonstrate the successful implementation of AI in logistics and route optimization. These case studies showcase the tangible benefits and positive impact that AI-powered systems can bring to logistics companies. Some examples include:
1. A global logistics provider, implemented an AI-powered route optimization system that analyzed real-time traffic data, weather conditions, and delivery demands. As a result, they achieved a 15% reduction in fuel consumption, a 20% decrease in delivery time, and improved customer satisfaction.
2. A regional courier service, utilized AI algorithms to optimize their delivery routes based on vehicle capacity, traffic patterns, and customer preferences. This enabled them to reduce the number of vehicles on the road, decrease operational costs by 10%, and provide faster delivery services.
These case studies highlight the transformative impact of AI in logistics, demonstrating its potential to drive significant improvements in efficiency, cost savings, and customer satisfaction.
Future Trends in AI-driven Route Optimization
The future of AI-driven route optimization in logistics holds immense potential for further advancements and innovations. Some of the key trends to watch out for include:
1. Integration with IoT: The integration of AI with the Internet of Things (IoT) enables logistics companies to gather real-time data from sensors and devices throughout the supply chain network. This data can be used to optimize routes, monitor vehicle conditions, and enhance overall logistics operations.
2. Predictive Analytics: AI-powered route optimization systems can leverage predictive analytics to anticipate demand patterns, identify potential bottlenecks, and proactively optimize routes. By analyzing historical data and trends, logistics companies can make data-driven decisions to improve efficiency and reduce costs.
3. Autonomous Vehicles: The rise of autonomous vehicles presents new opportunities for AI-driven route optimization. Self-driving vehicles can leverage AI algorithms to navigate efficiently, adapt to real-time traffic conditions, and optimize their routes in real-time. This can lead to further cost savings and increased efficiency in logistics operations.
4. Collaborative Optimization: AI-driven route optimization can facilitate collaboration between multiple logistics companies, enabling them to share resources, consolidate shipments, and optimize delivery routes collectively. This collaborative approach can lead to significant efficiency gains and cost savings for all participating companies.
As AI technology continues to evolve, these future trends in route optimization hold promise for transforming the logistics industry and shaping the future of supply chain operations.
Are you looking for a trustworthy technology partner for GenAI implementation?
FindErnest empowers businesses with AI-driven and innovative digital solutions tailored to enhance business performance. Leveraging GEN AI, our agile and strategic approach provides:
-
Accelerated Time-to-Market: Reduced go-to-market cycles, enabling faster response to market demands.
-
Increased Productivity: Optimized workflows and automated processes, enhancing efficiency and output.
-
Improved Customer Experience: Tailored solutions that meet unique customer needs, delivering tailored products and services.
-
Data-Driven Insights: Advanced data analytics and reporting, providing critical insights to inform decision-making.
-
Enhanced Performance: Improved operational efficiency, reduced costs, and increased revenue growth.
-
Uncompromising Security: Robust security measures to safeguard data and assets.
-
Expert Support and Implementation: Dedicated support teams for seamless implementation and ongoing maintenance.
-
Data Warehousing and Datalake Management: Centralized data repository for comprehensive data analysis and insights.
Generative AI has the potential to revolutionize the logistics and route optimization industry. It can ensure timely delivery while maximizing fuel and cost efficiency, and adapt dynamically to unforeseen challenges. By streamlining operations, logistics companies can enhance their competitive advantage and outperform their rivals. Recent advancements and a more natural language interface make it much easier to use.
Get in touch to discover the transformative potential of our Generative AI services!
Follow us on LinkedIn to never miss an update.
Learn how FindErnest is making a difference in the world of business