Data Engineering | Business Intelligence | Data Analytics | Data | Data Mapping | Data Patterns | Data Security | Databricks
FindErnest: Top Synthetic Data Company to Transform Your Data Strategy
Read Time 11 mins | Written by: Praveen Gundala
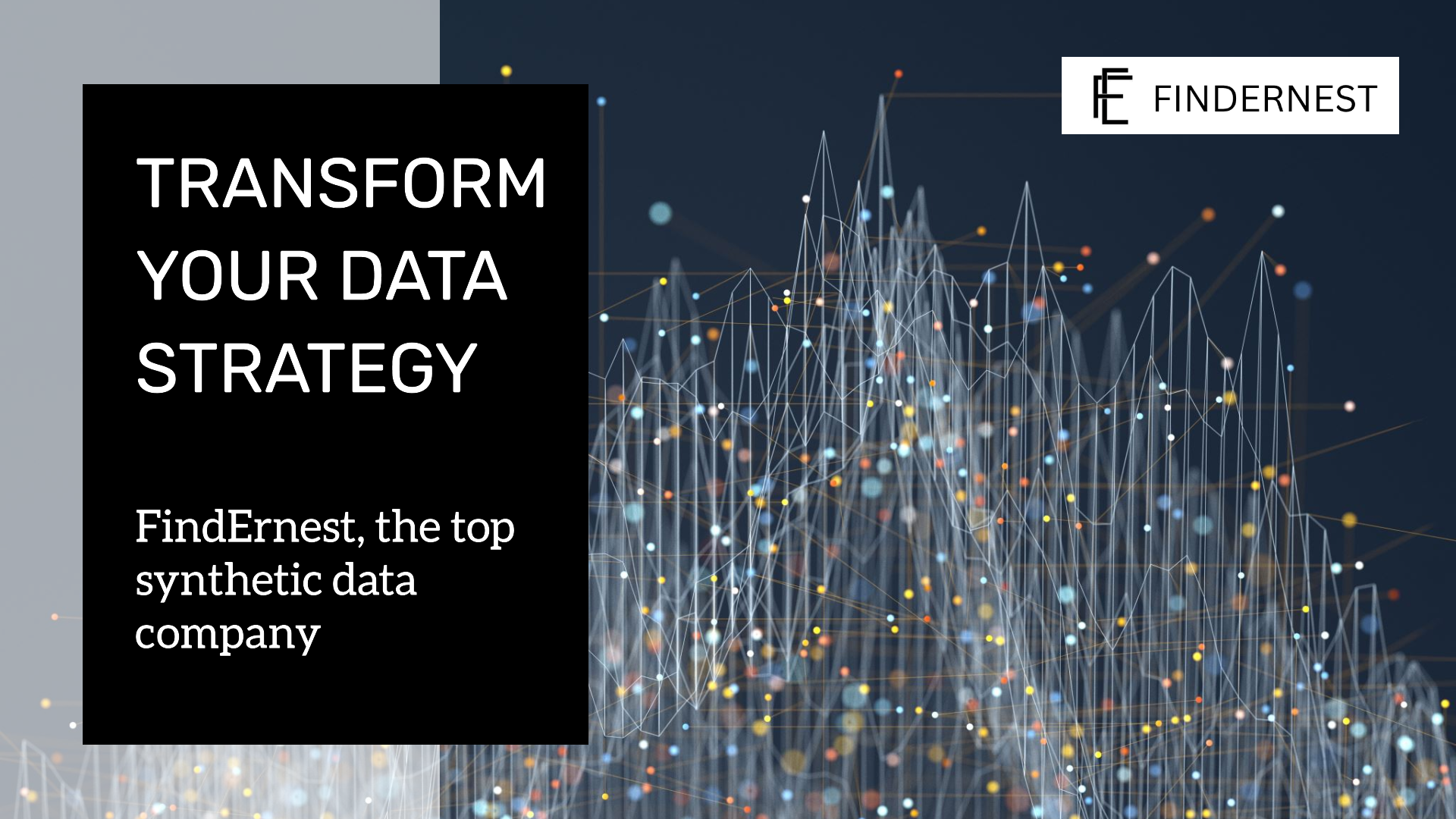
Discover the leading synthetic data companies that can revolutionize your data strategy and help you achieve your business goals. Selecting a synthetic data company like Findernest involves assessing your specific needs against the capabilities of potential partners. Focus on their technological offerings, support structures, compliance measures, and overall alignment with your organizational objectives. By doing so, you can ensure a fruitful long-term partnership that enhances your data-driven initiatives.
Understanding the Importance of Synthetic Data
Synthetic data generation involves creating artificial data that closely resembles the statistical characteristics and structure of real data. Companies utilizing synthetic data can skip the need for extensive data masking processes to safeguard sensitive or Personally Identifiable Information (PII).
Synthetic data serves as a secure alternative to actual production data and can significantly speed up testing and development, allowing companies to operate more flexibly without endangering user privacy.
For businesses aiming to enhance their artificial intelligence and machine learning models, synthetic data is a powerful asset. By producing data that imitates real-world data, organizations can tackle issues related to data privacy, scarcity, and bias.
The significance of synthetic data is in its ability to offer a diverse and comprehensive dataset that boosts model accuracy and reliability. It enables businesses to test various scenarios without risking sensitive information, ensuring adherence to data protection laws while still obtaining valuable insights.
Evaluating Features and Capabilities
When choosing a synthetic data company, it is crucial to evaluate the features and capabilities they offer. Key aspects to consider include the quality and realism of the synthetic data, the ease of integration with your existing systems, and the range of customization options available.
Additionally, it is important to assess the company's expertise in your industry and its ability to generate data that meets your specific needs. Look for features such as data anonymization, scalability, and support for various data types to ensure the solution aligns with your business goals.
Categories of Synthetic Data
Synthetic data can be categorized into three primary types:
1. Dummy/Mock (Human-Engineered) Data: This type of data is manually created to simulate real-world data. It's often used in software testing and development to create predictable, controlled scenarios. Although useful for initial testing phases, human-engineered data can lack the complexity and variability needed for advanced analytics and machine learning.
2. Simulation (Physics-Based) Data: Generated through simulations based on physical models and equations, this data type is prevalent in industries like automotive, aerospace and healthcare. For instance, simulations of crash tests or the human body's physiological responses can produce data that would be difficult, expensive or unethical to collect in real life.
3. Data-Driven (AI-Generated) Synthetic Data: This data is produced by algorithms and machine learning models, either pre-trained or trained on proprietary data. AI-generated synthetic data can closely mimic the statistical properties of real-world data, making it highly valuable for a wide range of applications, from training machine learning models to augmenting datasets for analytics.
AI-Generated Synthetic Data: Pre-Trained Models (LLMs) Vs. Generative Models
As enterprises increasingly adopt synthetic data, understanding the distinctions between data generated by pre-trained models like GPT (generative pre-trained transformer) and generative models trained on proprietary data is crucial. These differences can significantly impact data quality, privacy and usability.
Pre-Trained Models (LLMs)
Pre-trained models like GPT are built on vast datasets encompassing diverse domains and languages. These models excel in generating human-like text and are highly effective in scenarios requiring general-purpose data generation.
Their advantages include:
• Versatility: Pre-trained models can generate data across various domains without requiring domain-specific training.
• Privacy Assurance: Because pre-trained models don't use proprietary data, they also don't pose privacy concerns related to sensitive information.
• Customization Capabilities: These models can be fine-tuned and adapted to specific enterprise needs, offering a high degree of flexibility. However, this is complex, costly and can increase the risk of privacy exposure.
Their disadvantages include:
• Cost-Effectiveness: Utilizing pre-trained models can be expensive, especially when licensing fees or computational resources are considered.
• Data Quality: Pre-trained models lack the knowledge of a company's proprietary data. Hence, the generated data won't mimic the real-world behaviour of the organizations' data.
Generative Models Trained On Proprietary Data
Custom models, developed using an organization's unique datasets, provide a personalized method for synthetic data creation similar to Findernest. These models are crafted to comprehend and mirror the complexities of proprietary data, ensuring high accuracy and pertinence.
Their advantages include:
• Domain Specificity: Proprietary models are fine-tuned to an organization’s unique datasets, producing highly relevant and accurate synthetic data.
• Privacy Control: Although proprietary models are trained on real data, they can offer privacy controls to manage sensitive information appropriately.
• Cost Efficiency: Contrary to common belief, small generative models can be cost-efficient, as they eliminate the need for extensive data collection and labeling.
• Ease Of Maintenance: These models aren't necessarily resource-intensive or difficult to maintain, especially when acquired from specialized vendors.
Their disadvantages include:
• Complexity: The technology behind proprietary models is complex, making it challenging for enterprises to develop these models in-house.
• Vendor Dependency: Due to the complexity, enterprises may need to rely on vendors to provide these models, which can introduce dependencies.
What are the key data generation techniques to look for in a synthetic data company?
When evaluating synthetic data companies, it's essential to understand the various data generation techniques they employ. Here are the key methods to consider:
Key Data Generation Techniques
1. Generative Adversarial Networks (GANs)
GANs consist of two neural networks: a generator that creates synthetic data and a discriminator that evaluates its authenticity. This competitive process results in highly realistic synthetic datasets that can be used for various applications, including image and text generation2. Variational Autoencoders (VAEs)
VAEs compress the original data into a lower-dimensional space and then reconstruct it, generating synthetic data that closely resembles the original dataset. This technique is particularly effective in maintaining statistical properties while allowing flexibility in data generation3. Statistical Models
Techniques such as Gaussian Copulas or mixture models leverage the statistical properties of the original data to create synthetic datasets. These methods are useful for generating data that follows specific distributions, ensuring that the synthetic data retains similar characteristics to real-world data4. Rule-Based Generation
This approach involves creating synthetic data based on predefined rules or heuristics tailored to specific business needs. For example, healthcare datasets can be generated by applying rules related to demographics or medical conditions, ensuring relevance and utility5. Data Augmentation Techniques
Commonly used in fields like computer vision, these techniques involve transforming existing datasets through methods such as rotation, scaling, or adding noise to create new samples. This approach helps enhance the diversity of training datasets6. Agent-Based Models
These models simulate individual entities' behaviours and interactions within a system, generating synthetic data that reflect complex dynamics observed in real-world scenarios. They are particularly useful for applications requiring detailed behavioural insights7. Random Sampling and Interpolation
Random sampling involves generating new data points by selecting randomly from existing datasets, while interpolation creates new points by estimating values between known data points. Both methods can enhance dataset size and diversity
8. Hybrid Approaches
Combining real data with theoretical distributions allows for the generation of hybrid datasets. This method is advantageous when only partial real data is available, ensuring that the synthetic dataset retains relevant characteristics
The choice of synthetic data generation technique depends on factors such as the type of data needed, privacy considerations, and the specific requirements of your application. Understanding these methods will help you select the right tools for effective synthetic data generation tailored to your needs.
Cost Versus Value: Making an Informed Decision
Cost is always a significant factor when selecting a synthetic data company, but it should be weighed against the value the solution brings to your business. While some companies may offer lower prices, it is essential to consider the quality of the data and the level of support provided.
Investing in a higher-priced solution that delivers superior data quality and robust support can lead to better outcomes in the long run. Evaluate the return on investment by considering how the synthetic data will enhance your models, improve decision-making, and drive business growth.
Benefits of an End-to-End Synthetic Data Solution
An end-to-end synthetic data solution offers a comprehensive approach to generating, managing, and utilizing synthetic data throughout its lifecycle. Here are the key benefits of such a solution:
1. Unlimited Data Generation
An end-to-end solution allows organizations to generate synthetic data on demand and at scale, providing a virtually limitless supply tailored to specific needs. This capability is crucial for training machine learning models and conducting simulations without the constraints of real-world data availability2. Enhanced Privacy and Security
By using synthetic data, organizations can mitigate privacy concerns associated with real data. The generated datasets do not contain personally identifiable information (PII), allowing for compliance with stringent data protection regulations while still enabling robust analytics and research3. Cost-Effectiveness
Generating synthetic data can be more cost-effective than collecting and labeling real-world data, especially in industries where data acquisition is labor-intensive or requires specialized equipment. This cost efficiency extends to both the generation process and the reduced need for extensive data management4. Improved Data Quality and Variety
End-to-end solutions can produce high-quality synthetic datasets that address issues like class imbalance and missing values, enhancing the overall quality of machine learning models. Additionally, these solutions can introduce greater diversity into datasets, helping to reduce bias and improve fairness in AI applications5. Faster Development Cycles
With the ability to generate synthetic data quickly, organizations can accelerate their development workflows. This speed is particularly beneficial for testing new features, validating algorithms, and conducting virtual experiments without waiting for access to real production data6. Flexibility and Control
An end-to-end solution provides organizations with greater control over the quality, format, and characteristics of the synthetic datasets produced. Users can customize parameters based on specific project requirements, ensuring that the generated data meets their exact needs7. Risk Reduction
Utilizing synthetic data helps mitigate risks associated with using sensitive real-world data, such as potential breaches or compliance violations. This risk reduction allows organizations to innovate more freely while maintaining high standards of data governance8. Facilitation of Collaboration
Synthetic data can be shared more freely among teams and across organizations since it does not contain sensitive information. This capability fosters collaboration and knowledge sharing while maintaining privacy standardsAn end-to-end synthetic data solution empowers organizations to leverage high-quality, diverse datasets while addressing privacy concerns and reducing costs. By integrating all aspects of synthetic data management—from generation to application—these solutions enable faster innovation cycles and more effective use of AI technologies across various domains.
Selecting the Optimal Synthetic Data Provider like Findernest
Choosing the right synthetic data company, such as Findernest, requires careful consideration of several factors to ensure that the partnership aligns with your organization's specific needs and goals. Here are key aspects to evaluate:
Key Considerations for Choosing a Synthetic Data Company
1. Data Generation Techniques
Prioritize companies that utilize multiple data generation techniques, including Generative AI, which can create synthetic datasets that mimic the statistical properties of real data while ensuring privacy and compliance.
Look for features such as:
- Data masking and noise addition to protect sensitive information.
- Techniques that maintain relational integrity to ensure data consistency.
2. Data Quality and Utility
Assess the company's ability to produce high-quality synthetic data that retains the patterns and distributions of the original datasets. This is crucial for applications in machine learning and analytics. Ensure that:- The generated data preserves referential integrity.
- There is a focus on maximizing data utility for practical applications.
3. Ease of Use
A user-friendly interface is essential, especially if your team lacks extensive coding expertise. Look for platforms that offer:- Drag-and-drop features for easy data generation.
- Integration capabilities with existing IT infrastructure to minimize disruptions
4. Support and Training
Evaluate the level of support provided by the company. This includes:- Access to detailed manuals and training resources.
- Availability of technical support to assist with implementation and troubleshooting
5. Compliance and Security
Ensure that the synthetic data provider adheres to relevant data privacy regulations and offers robust security measures. This is particularly important in industries like healthcare and finance, where compliance is criticalWhen selecting a synthetic data provider, it's imperative to evaluate their proficiency in these essential techniques. A thorough understanding of these approaches is crucial to guarantee that the synthetic data produced aligns with your organization's needs for authenticity, functionality, and adherence to privacy regulations. Choosing a company like Findernest requires matching your particular requirements with the strengths of potential collaborators. Concentrate on their technology solutions, support frameworks, compliance protocols, and overall fit with your organizational goals. This approach will help secure a successful long-term collaboration that bolsters your data-centric efforts.
Learn how FindErnest is making a difference in the world of business
Praveen Gundala
Praveen Gundala, Founder and Chief Executive Officer of FindErnest, provides value-added information technology and innovative digital solutions that enhance client business performance, accelerate time-to-market, increase productivity, and improve customer service. FindErnest offers end-to-end solutions tailored to clients' specific needs. Our persuasive tone emphasizes our dedication to producing outstanding outcomes and our capacity to use talent and technology to propel business success. I have a strong interest in using cutting-edge technology and creative solutions to fulfill the constantly changing needs of businesses. In order to keep up with the latest developments, I am always looking for ways to improve my knowledge and abilities. Fast-paced work environments are my favorite because they allow me to use my drive and entrepreneurial spirit to produce amazing results. My outstanding leadership and communication abilities enable me to inspire and encourage my team and create a successful culture.