Machine Learning | MLOps | LLM | Retrieval Augmented Generation (RAG) | Natural Language Processing | NLP
Boosting NLP Performance with Retrieval Augmented Generation
Read Time 9 mins | Written by: Praveen Gundala
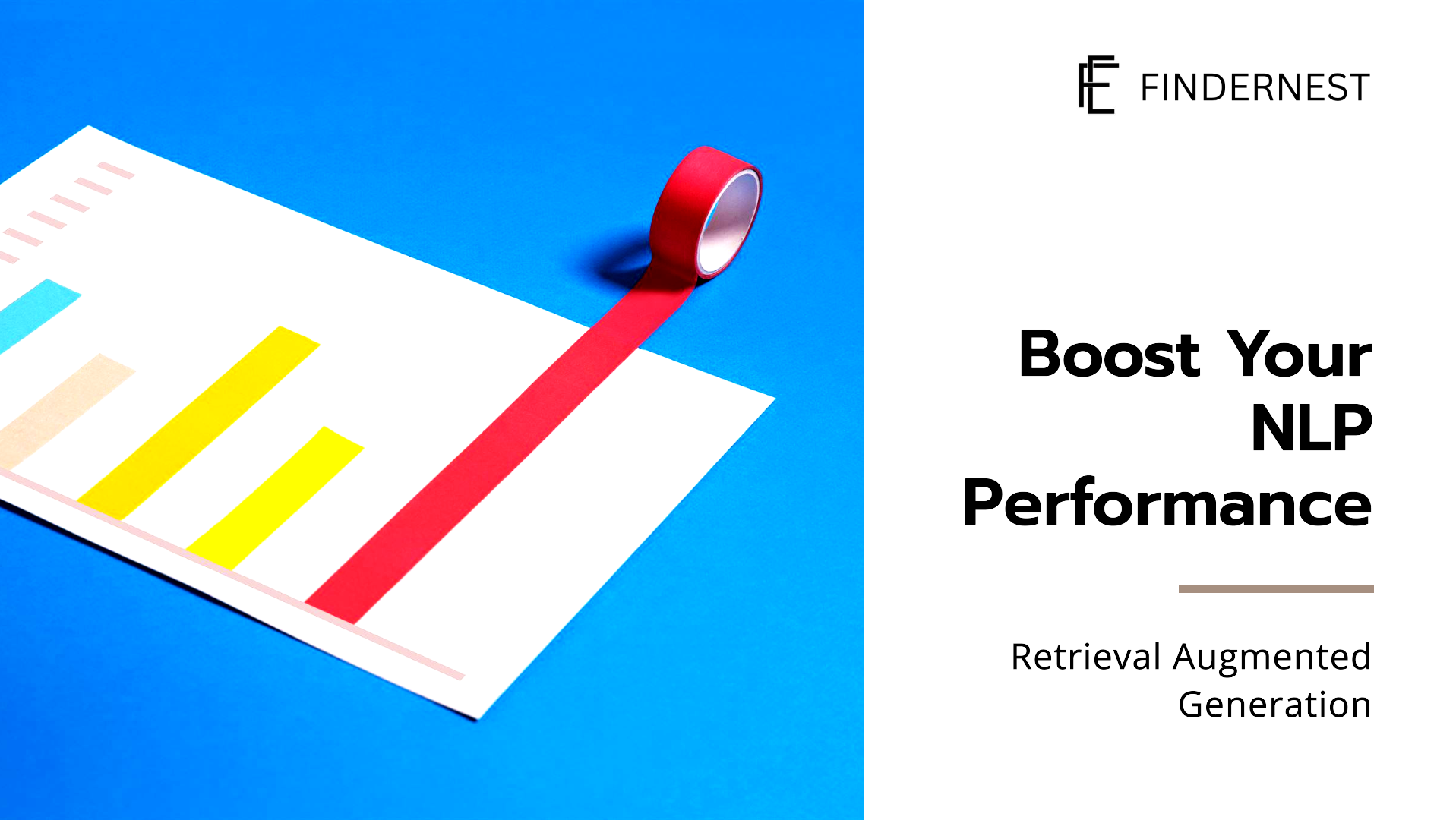
Discover how Retrieval Augmented Generation (RAG) is transforming the landscape of Natural Language Processing (NLP) by elevating performance and accuracy in data retrieval. RAG embodies the cutting-edge convergence of artificial intelligence and machine learning, harnessing the collective strengths of retrieval-based and generative models. These advanced systems not only showcase expanded knowledge but also exhibit exceptional responsiveness and adaptability.
Understanding Retrieval Augmented Generation (RAG)
Retrieval Augmented Generation (RAG) stands out as a groundbreaking method in Natural Language Processing (NLP) that merges the best attributes of retrieval-based techniques and generative models. This innovative concept involves extracting pertinent information from extensive datasets to craft responses that are not only more precise but also contextually relevant.
By harnessing pre-trained language models that are seamlessly integrated with external knowledge sources, RAG empowers systems to tap into a vast reservoir of information. This augmentation ensures that the content generated is not only pertinent but also enriched with the most up-to-date data.
NLP, a subset of artificial intelligence (AI), grants computers the ability to comprehend and decipher language, opening doors to a myriad of applications. From analyzing customer feedback to translating documents and generating marketing content, NLP has paved the way for the rise of large language models (LLMs) – AI tools renowned for their human-like text responses.
Despite the remarkable capabilities of NLP technologies like LLMs, even sophisticated models often struggle with specialized tasks. This is primarily due to three key limitations in NLP:
1. NLP systems are confined to the knowledge acquired during a finite training period, leading to a decrease in accuracy as training data becomes outdated.
2. Models may falter when faced with tasks beyond their foundational knowledge, resulting in unreliable outcomes.
3. Models are prone to improvising or generating irrelevant information due to knowledge gaps.
To surmount these limitations and uphold the accuracy and relevance of AI-generated content, businesses can embrace retrieval-augmented generation (RAG).
The Role of RAG in Enhancing NLP Capabilities
RAG significantly enhances NLP capabilities by improving the accuracy and relevance of information retrieval. Traditional NLP systems often struggle with understanding context or may generate responses that lack depth. RAG addresses these limitations by integrating a retrieval component that supplements the generative model with pertinent data.
This hybrid approach not only boosts the quality of responses but also expands the range of applications for NLP technologies. By facilitating more precise and context-aware interactions, RAG enables businesses to deliver superior customer experiences and make more informed decisions.
Traditional generative models can sometimes produce responses that lack relevance or accuracy. By incorporating retrieval-based methods, RAG can:
- Provide contextually accurate information.
- Reduce the generation of irrelevant or incorrect responses.
- Improve the overall performance of NLP applications.
Components of a RAG Model
A RAG model consists of two main components:
- Retrieval Component: This component retrieves relevant information from a large corpus of documents.
- Generation Component: This component generates text based on the retrieved information.
Key Technologies Behind RAG: From Machine Learning to Knowledge Bases
The core technologies driving RAG include advanced machine learning algorithms, large-scale language models, and comprehensive knowledge bases. Machine learning techniques are used to train models that can effectively retrieve and generate relevant information.
Knowledge bases play a crucial role by providing a structured repository of information that the retrieval component can access. This integration of machine learning and knowledge bases ensures that RAG systems are both intelligent and well-informed, capable of delivering nuanced and accurate outputs.
Challenges and considerations for integrating RAG in NLP
While RAG can significantly improve NLP functionality, success depends on an enterprise’s integration strategy. Organizations should consider factors like data quality and use case requirements before investing in RAG development.
Data quality
NLP performance relies heavily on the quality of data used to build a knowledge base. If this information is biased, irrelevant, incorrect, or outdated, the model will reflect this in its outputs, negating the original goal of improving accuracy.
Use case suitability
According to research on retrieval-augmented language models, RAG-enhanced NLP is not suitable for every use case. RAG is strongest in domain-intensive problems benefitting from factual information retrieval, especially in fields where current standards, practices, or insights evolve regularly. Tasks that rely on NLP’s creative strengths and core aspects of a model’s behaviour, like the writing style, tend to benefit less from RAG. For example, RAG can effectively support summarization tasks or question-answer systems, but it’s not as useful for achieving a specific conversational tone for a customer chatbot.
Development costs
One of the primary benefits of integrating RAG with NLP is its relatively low cost compared to fine-tuning. However, organizations will still need to consider the cost of retrieval architecture development as well as data management and storage, which can increase significantly with larger knowledge bases. Some use cases may also require a hybrid approach, combining RAG with fine-tuning, which can dramatically raise development costs.
Security
RAG introduces new security vulnerabilities to your AI systems. In particular, the technique’s retrieval phase is vulnerable to prompt-based attacks, in which attackers use cleverly worded queries to trick the model into revealing sensitive data. For example, attackers may use queries that cause a model to retrieve information similar in context or meaning to proprietary documents. This enables adversaries to get a sense of the private information stored in a knowledge base without needing to directly infiltrate the system.
Efficiency and scalability
With RAG applications, models take the additional step of retrieving knowledge base data before responding to user queries, resulting in a slightly slower system. Larger knowledge bases can delay this process further. While this may not be an issue for some applications, organizations should consider increased latency when using RAG for rapid information retrieval cases.
Real-World Applications of RAG in Various Industries
RAG is making a significant impact across various industries. In healthcare, it aids in retrieving and generating medical information, assisting doctors in diagnosing and treating patients more effectively. In finance, RAG enhances analytical tools, enabling more accurate market predictions and financial planning.
Retail and customer service sectors also benefit from RAG, as it powers chatbots and virtual assistants that provide personalized customer support. By leveraging RAG, businesses can automate and optimize interactions, leading to improved customer satisfaction and operational efficiency.
Who Needs NLP Consulting?
Virtually any organization that handles large amounts of text or needs to understand customer interactions can benefit from NLP consulting.
However, certain sectors may find these services particularly transformative
- Retail and E-Commerce: For these sectors, NLP can revolutionize customer interaction by providing personalized shopping experiences and improving customer service through chatbots and automated responses.
- Healthcare: NLP technologies can help manage patient records, extract relevant patient information, and support research by analyzing medical texts.
- Banking and Finance: In this industry, NLP is used to monitor compliance, analyze financial documents, and enhance customer service through automated systems that understand and process customer queries efficiently.
- Legal Services: Custom NLP consulting can aid in document review, legal research, and case preparation by swiftly analyzing large volumes of legal texts.
- Government: For public services, NLP can improve citizen engagement and streamline communication channels, making information access and government services more efficient.
NLP consulting isn't just for tech-savvy companies or large corporations. It's a strategic asset that can be customized and scaled to fit the needs of any organization that wants to enhance its operations through improved understanding and management of natural language data.
By partnering with NLP consulting companies, businesses across various sectors can unlock new opportunities for growth and efficiency, making NLP an indispensable part of modern business strategy.
Future Trends and Predictions for RAG in NLP
As RAG technology continues to evolve, we can expect to see even more sophisticated NLP applications. Future trends point towards deeper integration of RAG with other AI technologies, such as computer vision and speech recognition, creating more holistic and multi-modal AI systems.
Additionally, advancements in machine learning techniques and the expansion of knowledge bases will further enhance the capabilities of RAG systems. Businesses that adopt these cutting-edge technologies will be well-positioned to harness the full potential of NLP, driving innovation and gaining a competitive edge in their respective fields.
Ensuring success for your enterprise use case
RAG effectively addresses NLP limitations, enabling models to retrieve current, factual information without the time and resource commitments of fine-tuning. While RAG and NLP function well together, you must consider whether combining these techniques will improve your AI output reliability in practice.
For successful integration, investigate whether your use case is suitable for RAG and, if needed, combine RAG with techniques like fine-tuning to optimize NLP performance. As a first step in knowledge base development, gather only high-quality, accurate, and relevant data for your target task to ensure reliable results.
Use security best practices to protect your AI tools from common malicious attack methods like prompt injection. This includes strict user access controls for NLP systems, as well as sanitizing, encrypting, and backing up all knowledge base data. Invest in continuous monitoring and alerting solutions designed to detect anomalies unique to AI, such as suspicious prompts.
RAG is an important innovation in the field of AI, improving NLP reliability for a variety of domain-focused enterprise use cases, from analyzing financial data with current market trends to supporting customer chatbots with the latest product documentation. To maintain optimal performance, stay up-to-date with emerging techniques and adapt your RAG application based on the latest best practices.
Navigating the labyrinth of Natural Language Processing (NLP) can be intimidating for businesses seeking to harness its power for better customer interactions, streamlined processes, or enhanced data analysis.
Whether you're a small enterprise dipping your toes in NLP waters or a larger corporation looking to refine your approach, understanding the process from start to finish is crucial.
Define your NLP goals
Embarking on an NLP consulting journey starts with defining clear objectives. Before delving into the technical aspects, it's crucial to pinpoint your desired outcomes with NLP.
These goals can vary from enhancing customer service through chatbots and extracting valuable insights from customer feedback to streamlining repetitive tasks.
Having a specific target not only acts as a guiding light for the path ahead but also provides a benchmark for measuring progress.
Data collection and analysis
After achieving your NLP goals, the next step is to gather and prepare your text data. This process involves collecting relevant samples such as customer queries, social media posts, or company reports. Once you have gathered the data, it undergoes a meticulous cleaning and formatting process to eliminate irrelevant pieces and ensure consistency. This crucial step serves as the foundation of your NLP project, as the quality of the data greatly impacts the outcomes, much like fertile soil nurtures a successful harvest.
NLP model development and training
Crafting the system is a delicate art, requiring a blend of technical expertise and creative finesse. Collaborating developers and data scientists unite to sculpt an NLP model tailored to meet your objectives. Delicately choosing the optimal algorithms and tools, potentially sourced from the top NLP Platforms for Businesses, they customize as needed before embarking on model training with the meticulously prepared data. This iterative process of teaching and learning allows the system to progressively refine its language comprehension and processing capabilities.
Testing and refinement
Ensuring precision and efficacy is the cornerstone of this phase. The developed NLP system undergoes rigorous testing to assess its performance with real-world data.
This critical stage focuses on rectifying any errors and optimizing the model to better align with the specified objectives. It's common for NLP consulting teams to revisit previous steps, making adjustments and enhancements before progressing further.
Integration and deployment
The final step is to implement the NLP system within your organization's framework. This involves seamlessly integrating the NLP solution into existing platforms or creating new interfaces as needed. The objective is to ensure a seamless integration that enhances operational efficiency without causing disruptions. Once operational, the value of NLP will be evident through improved customer experiences and streamlined internal processes.
Conclusion
RAG, or Retrieval-Augmented Generation, is a groundbreaking advancement in the field of NLP that seamlessly merges the strengths of retrieval-based and generative models. Implementing the steps outlined in this article will empower you to unleash the full potential of your RAG model, opening up a multitude of possibilities.
From inception to execution, FindErnest delivers impactful outcomes by blending cutting-edge technology with transformative growth strategies. Our tailored solutions tackle each challenge head-on, striving for tangible success alongside our clients. With services that enhance customer satisfaction, optimize operations, and provide valuable insights, we utilize efficient tools and strategies to drive exceptional results. Explore FindErnest’s array of innovative human capital solutions, spanning Technology Consulting, AI, Cybersecurity, Cloud, and Managed Services, to propel global enterprises towards success and innovation with unmatched service. As your Trusted Tech Partner, we unlock exponential growth through scalable solutions and seasoned technology teams, accelerating time to market, fostering digital transformation, and rapidly building MVPs.
In essence, RAG epitomizes the cutting-edge fusion of artificial intelligence and machine learning, harnessing the combined strengths of retrieval-based and generative models. These systems not only boast enhanced knowledge but also demonstrate remarkable responsiveness and adaptability.
As RAG continues to evolve, it holds the potential to enhance our daily interactions with technology, offering personalized and contextually relevant experiences. Despite encountering obstacles, the future of RAG appears promising, with a focus on seamless integration into our daily routines and improving decision-making processes.
Learn how FindErnest is making a difference in the world of business
Praveen Gundala
Praveen Gundala, Founder and Chief Executive Officer of FindErnest, provides value-added information technology and innovative digital solutions that enhance client business performance, accelerate time-to-market, increase productivity, and improve customer service. FindErnest offers end-to-end solutions tailored to clients' specific needs. Our persuasive tone emphasizes our dedication to producing outstanding outcomes and our capacity to use talent and technology to propel business success. I have a strong interest in using cutting-edge technology and creative solutions to fulfill the constantly changing needs of businesses. In order to keep up with the latest developments, I am always looking for ways to improve my knowledge and abilities. Fast-paced work environments are my favorite because they allow me to use my drive and entrepreneurial spirit to produce amazing results. My outstanding leadership and communication abilities enable me to inspire and encourage my team and create a successful culture.